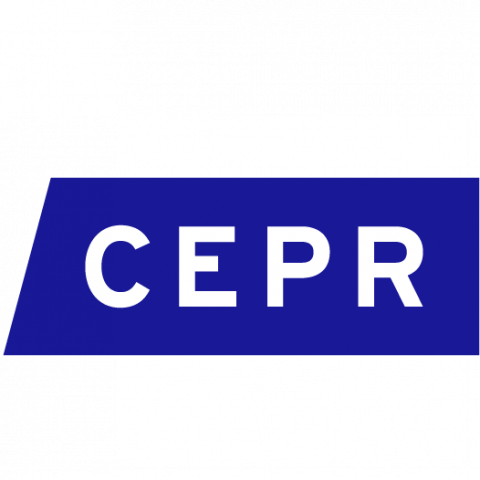
Machine learning and satellite data can help capture how households respond and adapt to floods. Providing better quality information can make climate change adaptation more efficient.
Read “Floods” and “Learning about a warming world: Attention and Adaptation in Agriculture” by Dev Patel.
A quarter of the world’s population is at flood risk, nearly 90% of whom live in poor countries (Rentschler et al. 2022). Dev Patel speaks to Tim Phillips in this episode of VoxDevTalks, building on his research measuring floods, their impacts, and the adaptation decisions of households.
Bangladesh as ground zero for climate change
Dev Patel focuses much of his research on Bangladesh, a country he argues is at the intersection between climate change and economic development.
‘The combination of density, poverty and vulnerable geography [made me] turn it into ground zero for climate changes’ most devastating impacts.’
- Around 170 million people live in the country, which is the size of the state of Georgia and this dense population means that any climate shock will impact many people.
- Despite achieving strong economic growth, Bangladesh is still a relatively poor country.
- Bangladesh sits on the front-line of climate change as it lies along the Ganges-Brahmaputra delta, the largest delta in the world. Much of the surrounding land is low and very flat making it vulnerable to floods and cyclones.
Existing data on flooding is biased
For his research, Dev Patel defines floods as unexpected inundations of land that is normally dry. Measuring the impact of floods is challenging given that much of the existing data can be biased. Hydrological models make very strong assumptions and predictions about future flooding and therefore may fail to account for the economic phenomenon called the ‘Lucas critique’ – an expectation of flooding in the future means individuals or households will implement protective strategies lowering their future flood risk.
There are two main ways that data on floods is collected.
- In situ, often measuring river height. However, there may be a scarcity of gauges in poor countries and river height measures may not be the best at capturing flood risk. Instead, flood coverage – a horizontal measure – is more appropriate.
- Data collected from news articles and government reports. This is problematic because floods in poor countries, particularly small, localised floods, are systematically underrepresented in such datasets.
The use of satellite data to fill potential data gaps may be constrained if data is measured conditional on knowing that a flood already occurred somewhere. The bias in flood data has direct policy consequences. A lack of sufficient local variation in flood risk hinders forecasting and the targeting of support.
Remote sensing models are also used to measure and predict flooding. However, in poor countries there may be very few data points on which these models can be trained leading to issues of reliability and forecasting (find out more about these issues in this VoxDevTalk by Tamma Carleton and Reed Walker).
Building new flood data
Dev Patel combines elements of machine learning and geophysics to build detailed flood data using two types of satellites: optical and radar. The former orbits the earth regularly but cannot capture imagery when it is very cloudy (often the case during flooding). Radar, on the other hand, can provide higher quality imagery (not obscured by clouds) by seeing how much radiation given off by the satellite is reflected back up from earth. However, these satellites orbit less frequently and therefore will not capture floods that only last a couple days.
When using data from these two satellites there is a trade-off between quantity and quality. To try and combine the advantages of both, Dev Patel, trains a machine learning algorithm to replicate the radar data using the optical measure. After starting with Bangladesh, he now aims to build a database that will cover the whole world.
Measuring the impact of floods in Bangladesh
Bangladesh has data on education, occupation and migration behaviour for around 19 million people over the past 20 years, including exact information on where they live. Patel combines this with satellite data of nighttime luminosity (acting as a proxy for economic activity) and physical space occupied (to capture the destruction of physical capital).
The flood data that Dev Patel constructs allows for more granular view of flooding. As there is substantial local variation in flood exposure, this more detailed data allows for the identification of potential control groups, which are essential for isolating a causal effect of floods.
He finds persistent negative impacts on nighttime light intensity up to seven years after a flood, as well as reductions in the physical space occupied. Flooding pushes workers out of agriculture, increases interhouse migration and leads to higher rates of school attendance, with the potential therefore to have long-run labour force participation or occupational impacts.
How do farmers in Bangladesh adapt to climate change?
His results also provide evidence on the climate change adaptation strategies of farmers (see also two other VoxDev articles on adaptation strategies via finance and irrigation). Farmers that experience more floods in the past experience fewer damages in future floods indicating that households learn from previous floods to cope with future floods.
The way in which households or individuals update their beliefs following a flood is important for adaptation decisions. As floods have significant agronomic impacts (particularly following tidal surges that change the salinity of the soil), it is important to understand how farmers update their beliefs following a flood and then manage their land. Dev Patel finds farmers both overestimate and underestimate salinity.
‘Farmers aren’t making any mistakes per se but rather just face a really difficult problem. Its due to the nature of environmental issues themselves – a lot of environmental risks are just difficult for us to observe and all we actually observe are the impacts of those dangers.’
As farmers often only observe outcomes it is hard to isolate which factor caused a bad harvest – pests, fungus, heat or salinity? To try and understand how farmers update their beliefs following a climate shock, Dev Patel uses a quasi-natural experiment and measures how farmers change their beliefs following two different types of shocks that lead to the same change in soil salinity but differ in how they are observed and thus how they change beliefs.
Experiencing the shock which is clearly observable leads to farmers updating their beliefs that the land is very salty and thus attributing bad harvests observed in the future as a result of the salt in the land. Interestingly, Patel’s results also indicate that it is more common for farmers to overestimate, as opposed to underestimate, soil salinity. This can have negative impacts given that climate change adaptation is costly; over adopting climate technology can be harmful.
‘In a place like Bangladesh that faces threats from heat, pests, floods, cyclones, salinity, all at the same time, it becomes incredibly challenging for people to accurately learn about each of those risks.’
Sharing environmental data with farmers can help adaptation to floods
A relatively cheap, simple and effective policy is to help farmers by collecting more environmental data and sharing it with them. Dev Patel reports that 80% of farmers are willing to pay at least a little bit for a salinity reading. The research also suggests that farmers do not care if it’s from their own plot, but value readings from villages around them as well.
References
Patel, D (2024), “Floods,” Working Paper.
Patel, D (2024), “Learning about a warming world: attention and adaptation in agriculture,” Working Paper.
Rentschler, J, M Salhab, and B A Jafino (2022), “Flood exposure and poverty in 188 countries,” Nature Communications, 13: 3527.